Deep dive into Predictive Maintenance Technology & Strategies
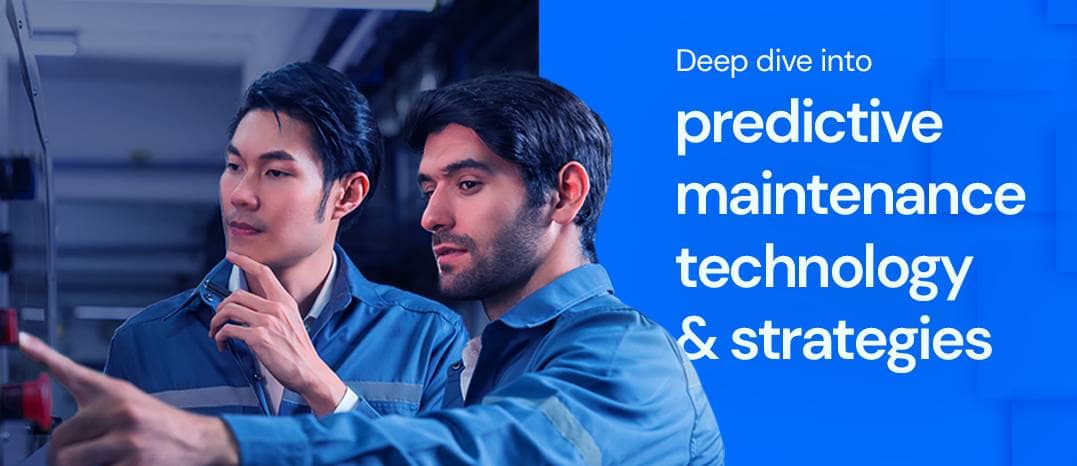
Introduction
Predictive Maintenance (PdM) revolutionizes industrial maintenance by leveraging technology and data to foresee equipment failures before they happen. This comprehensive exploration delves into predictive maintenance’s core principles, its components, the technology driving it, its benefits, and the challenges it faces. It sheds light on how Predictive maintenance transforms maintenance strategies from reactive to proactive, ensuring operational continuity and efficiency.
Understanding Predictive Maintenance
What is Predictive Maintenance?
Predictive Maintenance is a proactive maintenance strategy that harnesses advanced technologies and data analytics to predict equipment failures before they occur. Unlike traditional reactive or scheduled maintenance approaches, predictive Maintenance relies on analyzing real-time data and machine learning algorithms to anticipate potential issues and determine the optimal time for maintenance actions.
Principle of Predictive Maintenance
Predictive Maintenance, at its core, operates on several fundamental principles:
Condition Monitoring:Predictive Maintenance continuously gathers and monitors equipment data in real-time through sensors and IoT devices. This data is analyzed to detect any deviations from normal operating conditions.
Data Analytics & Machine Learning:By leveraging sophisticated analytics and machine learning algorithms, predictive maintenance processes vast volumes of data to identify patterns, trends, and anomalies that could indicate impending equipment failure.
Predictive Analysis:The primary objective of predictive maintenance is to forecast potential failures or performance degradation. Through predictive analysis, maintenance activities are strategically scheduled, optimizing resources and minimizing disruptions to operations.
Critical Components of Predictive Maintenance
Implementing an effective Predictive Maintenance strategy involves integrating several vital components that enable the prediction, analysis, and proactive management of equipment health.
1. Data Acquisition: Sensors, IoT Devices, Data Sources
Sensors and IoT Devices:These devices form the backbone of data acquisition in predictive maintenance. Various sensors collect real-time data on equipment performance, temperature, vibration, pressure, etc. These sensors are often connected to IoT devices, facilitating continuous data streaming from the equipment to the central monitoring systems.
Data Sources:Predictive Maintenance systems aggregate data from diverse sources such as Supervisory Control and Data Acquisition (SCADA) systems, Manufacturing Execution Systems (MES), Enterprise Resource Planning (ERP) systems, and other operational databases. This multidimensional data aggregation provides a comprehensive view of equipment health and operating parameters.
2. Data Processing: Analytics, Machine Learning Models
Big Data Analytics:The collected data undergoes extensive analysis using advanced analytics tools and techniques. This includes processing large volumes of data in real-time to identify patterns, trends, and correlations.
Machine Learning Models:Machine learning algorithms learn from historical data, predict future equipment behavior, and identify early signs of anomalies or potential failures. These models continuously evolve and improve as they ingest more data, enhancing the accuracy of predictions over time.
3. Actionable Insights: Predictions, Anomalies Detection
Predictive Analytics:Predictive Maintenance generates predictive insights that forecast potential equipment failures or performance degradation. These insights are based on the analysis of historical data and current equipment conditions. Predictive models predict when maintenance actions should be taken to prevent disruptions or failures.
Anomaly Detection:Predictive Maintenance systems constantly monitor equipment data for deviations from normal operating conditions. Identifying anomalies triggers alerts or notifications, allowing maintenance teams to investigate potential issues before they escalate.
Preventive Maintenance vs. Predictive Maintenance
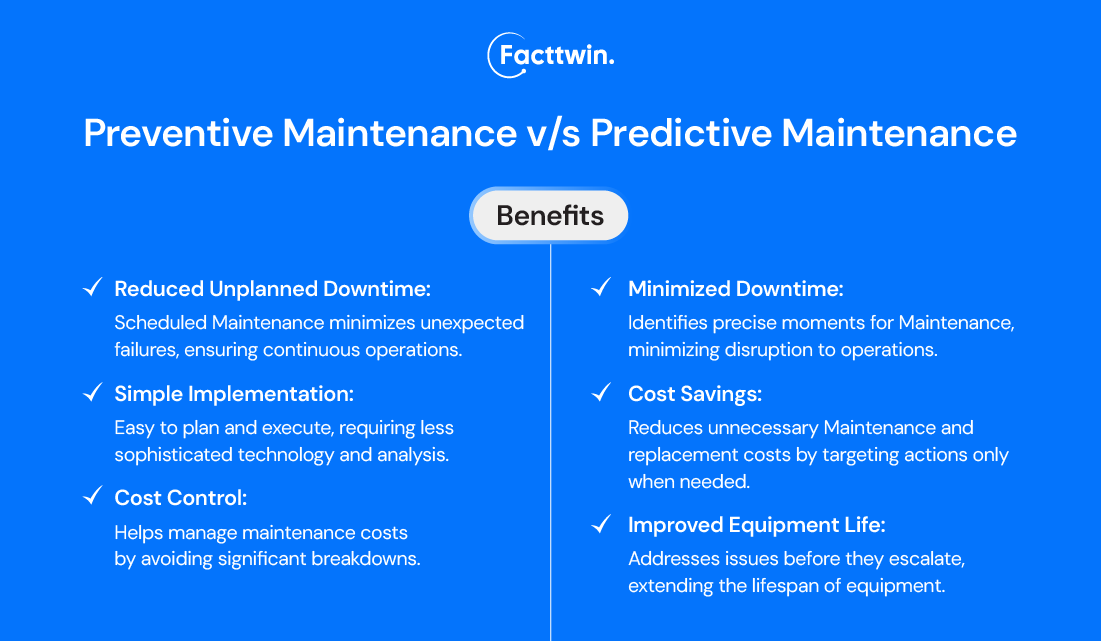
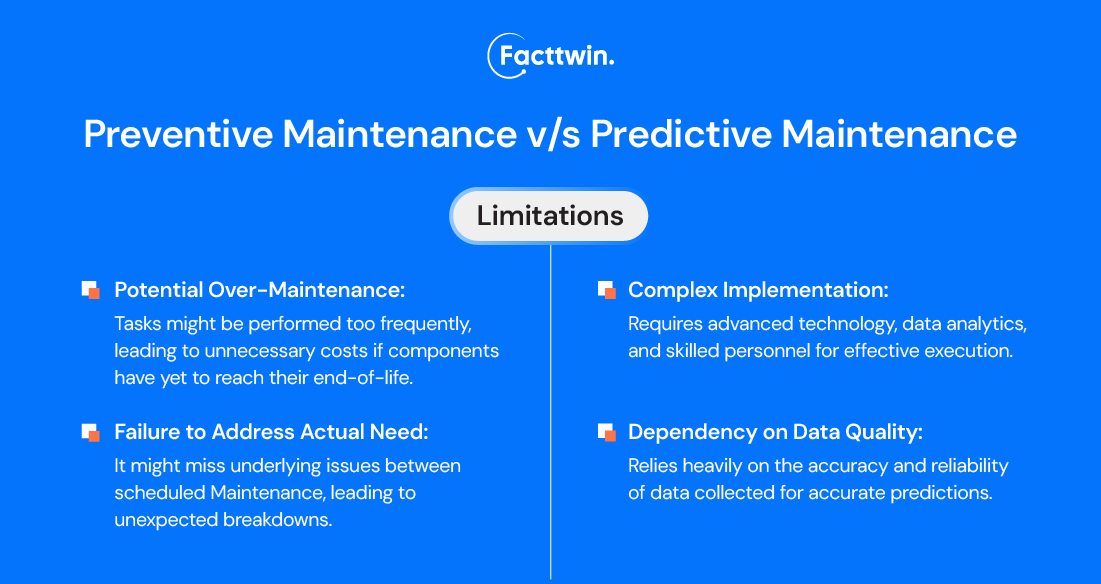
Preventive Maintenance:
Preventive Maintenance (PM) involves scheduled inspections and maintenance tasks performed at predetermined intervals, regardless of equipment condition.
Preventive Maintenance Approach:PM aims to prevent breakdowns by replacing components or servicing based on a fixed schedule. It follows a calendar-based or usage-based routine.
Benefits:
-
- Reduced Unplanned Downtime:Scheduled Maintenance minimizes unexpected failures, ensuring continuous operations.
- Simple Implementation:Easy to plan and execute, requiring less sophisticated technology and analysis.
- Cost Control:Helps manage maintenance costs by avoiding significant breakdowns.
Limitations:
-
-
- Potential Over-Maintenance:Tasks might be performed too frequently, leading to unnecessary costs if components have yet to reach their end-of-life.
- Failure to Address Actual Need:It might miss underlying issues between scheduled maintenance, leading to unexpected breakdowns.
-
Predictive Maintenance:
Predictive Maintenance (PdM) utilizes data-driven insights and analysis to predict equipment failure, allowing maintenance to be performed precisely when necessary.
Predictive Maintenance Approach:This uses real-time data from sensors and IoT devices to monitor equipment health. It employs analytics and machine learning to predict potential failures and prescribe maintenance actions.
Benefits:
-
-
- Minimized Downtime: Identifies precise moments for maintenance, minimizing disruption to operations.
- Cost Savings:Reduces unnecessary maintenance and replacement costs by targeting actions only when needed.
- Improved Equipment Life:Addresses issues before they escalate, extending the lifespan of equipment.
-
Limitations:
-
- Complex Implementation:Requires advanced technology, data analytics, and skilled personnel for effective execution.
- Dependency on Data Quality:Relies heavily on the accuracy and reliability of data collected for accurate predictions.
Who Uses Predictive Maintenance?
Predictive Maintenance is widely embraced across various industries and sectors where machinery, equipment, or assets play a critical role in operations. Some primary sectors and entities leveraging PdM include:
Manufacturing:Industries relying on machinery for production, such as automotive, aerospace, food processing, and pharmaceuticals, use predictive maintenance extensively to ensure continuous and reliable manufacturing processes.
Energy & Utilities:Power plants, utilities, and renewable energy facilities employ predictive maintenance to maintain crucial infrastructure, such as turbines, generators, and transmission equipment, optimizing performance and reliability.
Transportation & Logistics:From airlines using predictive maintenance for aircraft maintenance to shipping companies monitoring vessel engines, transportation sectors utilize predictive maintenance to enhance safety, efficiency, and reliability.
Healthcare:Hospitals and medical facilities utilize predictive Maintenance for critical equipment like MRI machines, X-ray equipment, and other medical devices to ensure they are always available for patient care.
Facility Management:Large commercial and residential buildings deploy predictive maintenance for elevators, HVAC systems, and other essential infrastructure to ensure uninterrupted operations and tenant comfort.
Benefits of Predictive Maintenance
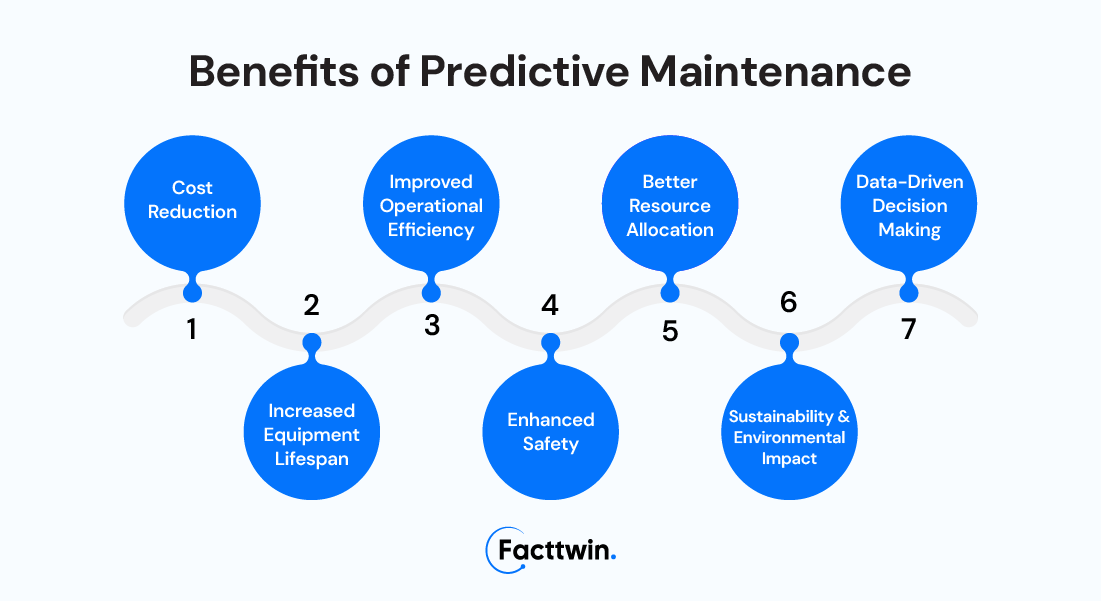
1. Cost Reduction:Predictive Maintenance minimizes unplanned downtime, reducing the costs associated with emergency repairs and production disruptions. It enables the optimization of maintenance resources, reducing overall maintenance expenditure.
2. Increased Equipment Lifespan:By identifying potential issues early, predictive maintenance helps address them before they lead to significant failures, extending the lifespan of machinery and assets.
3. Improved Operational Efficiency:Optimized maintenance schedules and reduced downtime enhance operational efficiency, improving throughput and productivity.
4. Enhanced Safety:Predictive Maintenance allows for identifying and mitigating safety risks associated with equipment failures, ensuring a safer working environment for employees.
5. Better Resource Allocation:By focusing maintenance efforts where they are most needed, predictive maintenance optimizes resource allocation, directing attention and resources to critical assets.
6. Data-Driven Decision Making:Predictive Maintenance generates a wealth of data that can be utilized for informed decision-making, process optimization, and strategic planning.
7. Sustainability & Environmental Impact:Predictive Maintenance helps reduce unnecessary waste generated by premature replacements or repairs, contributing to sustainability efforts.
Technologies Powering Predictive Maintenance
Predictive Maintenance harnesses advanced technology and data analytics to forecast equipment failures, ensuring proactive and timely maintenance actions. Integrating sensors, IoT devices, and cutting-edge analytics empowers industries to address potential issues, preemptively optimizing operational reliability and efficiency.
Sensors & Data Collection
Sensor Types:
- Vibration Sensors:Detect irregularities in rotating machinery.
- Temperature Sensors:Monitor heating/cooling issues in equipment.
- Pressure Sensors:Identify leaks or pressure-related failures.
- Acoustic Sensors:Detect abnormal sounds in machinery.
- Proximity Sensors:Monitor movement irregularities.
IoT Devices:
- Edge Computing Devices:Process data locally for faster analysis.
- Gateway Devices:Collect and transmit data to centralized systems.
Data Processing & Analytics in Predictive Maintenance
Big Data Analytics:
- Data Aggregation:Combines and cleans data for analysis.
- Pattern Recognition:Identifies trends and anomalies.
Machine Learning:
- Predictive Modeling:Learns from data to forecast failures.
- Continuous Improvement:Enhances predictions over time.
AI-Driven Decision Making:
- Automated Insights:Generates maintenance recommendations.
- Proactive Strategies:Predicts issues to prevent breakdowns.
Actionable Insights in Predictive Maintenance
Predictive Insights:
Predictive Maintenance generates invaluable insights by:
- Forecasting Failures:Predicting equipment issues before they occur based on data analysis and machine learning models.
- Optimal Maintenance Scheduling:Recommending precise times for maintenance actions to prevent potential breakdowns.
Anomalies Detection:
- Early Warning System:Identifies deviations from normal operating conditions, triggering alerts for potential issues.
- Proactive Measures:Enables investigation and intervention before problems escalate, minimizing downtime.
Advanced Strategies in Predictive Maintenance
- Failure Prediction & Prognostics- Prediction Maintenance extends beyond early detection to anticipate failure and optimize maintenance strategies.
- Early Warning Systems play a crucial role by analyzing patterns and deviations and flagging potential issues before they escalate. By recognizing abnormal behaviors in equipment, maintenance teams receive early alerts, enabling proactive interventions to prevent breakdowns.
- Prognostic Insights elevate maintenance planning by estimating assets’ Remaining Useful Life (RUL). Organizations can strategically schedule replacements or repairs by forecasting how much longer equipment will operate reliably, optimizing resource allocation.
- Prescriptive Maintenance Approaches- Moving from predictive to prescriptive maintenance involves leveraging insights for strategic action.
- Recommendations and action plans based on predictive analytics enable proactive measures, ensuring timely interventions before equipment failure. These recommendations guide maintenance teams in executing preventive actions precisely when needed.
- Optimization Strategies revolve around minimizing downtime and maximizing efficiency. Leveraging predictive insights, organizations refine maintenance schedules to minimize disruptions while enhancing overall equipment effectiveness.
- Integration with Maintenance Workflow- Successfully integrating Predictive Maintenance into existing workflows and harnessing human-machine collaboration is critical.
- Incorporating preventive maintenance seamlessly into current maintenance practices requires alignment with existing workflows. This integration streamlines operations, ensuring the adoption of predictive insights into day-to-day activities.
- Human-machine collaboration thrives by merging technician expertise with predictive analytics. Armed with data-driven insights, maintenance teams make informed decisions, combining their experience with advanced technologies to optimize maintenance activities.
Challenges & Future Trends in Predictive Maintenance
Implementing Predictive Maintenance faces several hurdles that organizations must address.
Data Quality & Integrity Issues:Ensuring the accuracy and reliability of data collected from various sensors and sources is critical. Data quality can lead to accurate predictions, impacting maintenance decisions.
Integration with Legacy Systems:Retrofitting existing infrastructure with predictive capabilities poses challenges. Integrating new technologies with older systems requires careful planning and compatibility considerations.
Skill Gaps & Training Needs:Adopting predictive maintenance necessitates a skill-set shift. Organizations must invest in training to equip their workforce with data analytics and machine learning competencies.
Emerging trends innovations:The future of Predictive Maintenance is poised for transformative advancements.
Edge Computing Advancements:The evolution of edge computing brings processing power closer to data sources, enabling faster analysis and real-time decision-making and reducing latency in predicting equipment failures.
AI Advancements:Explainable AI and Autonomous Decision-Making empower organizations to comprehend and trust AI-driven insights. Explainable AI clarifies AI reasoning, while autonomous decision-making allows AI systems to make maintenance decisions independently.
Predictive Maintenance as a Service (PdMaaS):A rising trend where third-party providers offer predictive maintenance solutions. This model allows companies to access advanced predictive capabilities without substantial infrastructure investments.
Learn more about how Facttwin can help you maximize your machine’s uptime Machine Health Monitoring & predictive maintenance.
Conclusion
Predictive Maintenance heralds a new era in maintenance practices, ushering in proactive, data-powered methodologies that minimize downtime and optimize resources. Embracing emerging trends like Predictive Maintenance as a Service, advancements in edge computing, and AI-driven decision-making promises a future where equipment reliability and operational excellence prevail. Predictive Maintenance isn’t just an upgrade; it’s a commitment to efficiency, sustainability, and seamless operations.
FAQ
1. What are the Three Types of Predictive Maintenance?
Vibration Analysis:Monitoring machinery vibrations to detect abnormalities or potential failures.
Infrared Thermography:Identifying irregular temperature patterns in equipment, indicating issues.
Oil Analysis:Examining lubricants for signs of wear or contamination in machinery.
2. What is Prescriptive Maintenance?
Prescriptive Maintenance refers to a more advanced stage beyond predictive Maintenance. It predicts when equipment failures might occur and prescribes the best course of action or recommendations to prevent those failures proactively.
3. What is the Predictive Maintenance Principle?
The primary principle of Predictive Maintenance revolves around leveraging data analytics, machine learning, and real-time monitoring to anticipate potential equipment failures and analyzing patterns, anomalies, and historical data forecasts when maintenance actions should be taken, optimizing resources and minimizing disruptions.
4. What is the Difference between Predictive and Preventive Maintenance?
Predictive Maintenance (PdM) anticipates equipment failures by analyzing real-time data and forecasts maintenance needs precisely when necessary. It aims to prevent failures before they occur.
Preventive Maintenance (PM) follows scheduled inspections or tasks at fixed intervals regardless of equipment condition. It prevents breakdowns by replacing parts or servicing based on a calendar or usage routine.
Advanced Strategies in Predictive Maintenance
- Failure Prediction & Prognostics- Prediction Maintenance extends beyond early detection to anticipate failure and optimize maintenance strategies.
- Early Warning Systems play a crucial role by analyzing patterns and deviations and flagging potential issues before they escalate. By recognizing abnormal behaviors in equipment, maintenance teams receive early alerts, enabling proactive interventions to prevent breakdowns.
- Prognostic Insights elevate maintenance planning by estimating assets’ Remaining Useful Life (RUL). Organizations can strategically schedule replacements or repairs by forecasting how much longer equipment will operate reliably, optimizing resource allocation.
- Prescriptive Maintenance Approaches- Moving from predictive to prescriptive maintenance involves leveraging insights for strategic action.
- Recommendations and action plans based on predictive analytics enable proactive measures, ensuring timely interventions before equipment failure. These recommendations guide maintenance teams in executing preventive actions precisely when needed.
- Optimization Strategies revolve around minimizing downtime and maximizing efficiency. Leveraging predictive insights, organizations refine maintenance schedules to minimize disruptions while enhancing overall equipment effectiveness.
- Integration with Maintenance Workflow- Successfully integrating Predictive Maintenance into existing workflows and harnessing human-machine collaboration is critical.
- Incorporating preventive maintenance seamlessly into current maintenance practices requires alignment with existing workflows. This integration streamlines operations, ensuring the adoption of predictive insights into day-to-day activities.
- Human-machine collaboration thrives by merging technician expertise with predictive analytics. Armed with data-driven insights, maintenance teams make informed decisions, combining their experience with advanced technologies to optimize maintenance activities.
Challenges & Future Trends in Predictive Maintenance
Implementing Predictive Maintenance faces several hurdles that organizations must address.
Data Quality & Integrity Issues:Ensuring the accuracy and reliability of data collected from various sensors and sources is critical. Data quality can lead to accurate predictions, impacting maintenance decisions.
Integration with Legacy Systems:Retrofitting existing infrastructure with predictive capabilities poses challenges. Integrating new technologies with older systems requires careful planning and compatibility considerations.
Skill Gaps & Training Needs:Adopting predictive maintenance necessitates a skill-set shift. Organizations must invest in training to equip their workforce with data analytics and machine learning competencies.
Emerging trends innovations:The future of Predictive Maintenance is poised for transformative advancements.
Edge Computing Advancements:The evolution of edge computing brings processing power closer to data sources, enabling faster analysis and real-time decision-making and reducing latency in predicting equipment failures.
AI Advancements:Explainable AI and Autonomous Decision-Making empower organizations to comprehend and trust AI-driven insights. Explainable AI clarifies AI reasoning, while autonomous decision-making allows AI systems to make maintenance decisions independently.
Predictive Maintenance as a Service (PdMaaS):A rising trend where third-party providers offer predictive maintenance solutions. This model allows companies to access advanced predictive capabilities without substantial infrastructure investments.
Learn more about how Facttwin can help you maximize your machine’s uptimeMachine Health Monitoring & predictive maintenance.
Conclusion
Predictive Maintenance heralds a new era in maintenance practices, ushering in proactive, data-powered methodologies that minimize downtime and optimize resources. Embracing emerging trends like Predictive Maintenance as a Service, advancements in edge computing, and AI-driven decision-making promises a future where equipment reliability and operational excellence prevail. Predictive Maintenance isn’t just an upgrade; it’s a commitment to efficiency, sustainability, and seamless operations.
FAQ
1. What are the Three Types of Predictive Maintenance?
Vibration Analysis:Monitoring machinery vibrations to detect abnormalities or potential failures.
Infrared Thermography:Identifying irregular temperature patterns in equipment, indicating issues.
Oil Analysis:Examining lubricants for signs of wear or contamination in machinery.
2. What is Prescriptive Maintenance?
Prescriptive Maintenance refers to a more advanced stage beyond predictive Maintenance. It predicts when equipment failures might occur and prescribes the best course of action or recommendations to prevent those failures proactively.
3. What is the Predictive Maintenance Principle?
The primary principle of Predictive Maintenance revolves around leveraging data analytics, machine learning, and real-time monitoring to anticipate potential equipment failures and analyzing patterns, anomalies, and historical data forecasts when maintenance actions should be taken, optimizing resources and minimizing disruptions.
4. What is the Difference between Predictive and Preventive Maintenance?
Predictive Maintenance (PdM) anticipates equipment failures by analyzing real-time data and forecasts maintenance needs precisely when necessary. It aims to prevent failures before they occur.
Preventive Maintenance (PM) follows scheduled inspections or tasks at fixed intervals regardless of equipment condition. It prevents breakdowns by replacing parts or servicing based on a calendar or usage routine.